The balance between “Know-Being” and “Know-How” : keys to motivation and entrepreneurial posture through an original data analysis on the dynamics of entrepreneurship and the impacts on the structures accompanying these entrepreneurs.
Authors:
Xavier Delaunay - Pepinium - https://www.pepinium.fr
Benoit Riou - Stat & More - https://statandmore.com
Date: 25 October 2023
Summary:
Entrepreneurship is an exciting journey, but fraught with complex challenges. This study explores the relationships between “Know-Being” and “Know-How” among potential contractors to better understand the elements underlying the motivation and development of entrepreneurial skills.
Through a Principal Component Analysis (PCA), an Ascending Hierarchical Classification (HCA), and a Bayesian analysis, we reveal the crucial importance of “Know-Being” in entrepreneurial motivation and success. The five groups of respondents identified by the HCA highlight the diversity of profiles among potential contractors, while the causal network using Bayesian analysis reveals complex relationships between the skills of “Know-Being” and “Know-How”. These emphasize the impact of behavior on motivation and entrepreneurial skills, reinforcing the need for a comprehensive approach in entrepreneurial coaching.
This study offers crucial perspectives for entrepreneurial support professionals, educators and decision-makers, highlighting the importance of cultivating both “Know-Being” and “Know-How” to foster entrepreneurial success.
Keywords:
Entrepreneurship, Soft Skills, Know-Being, Know-How, Motivation, Entrepreneurial Skills, Entrepreneurial Support, Principal Component Analysis, Hierarchical Classification Analysis, Bayesian Analysis, Causal Network
Key Ideas:
In the world of entrepreneurship, the key to success lies in the balance between “Know-Being” and “Know-How”. This study demonstrates that, before “Know-How”, it is essential to “Know-Being”. Behavioural and identity skills play a leading role in motivating and developing entrepreneurial skills. By exploring the complex relationships between these dimensions, we offer perspectives that we consider fundamental for professionals in entrepreneurial coaching, educators and decision-makers, highlighting the need for a comprehensive approach to support entrepreneurial success.
Introduction:
The world of entrepreneurship is fertile ground for innovation and economic growth. However, the path to entrepreneurial success is strewn with challenges and uncertainties. To better understand the elements underlying the motivation and development of entrepreneurial skills, it is essential to explore the complex relationships between “Know-Being” and “Know-How” among potential entrepreneurs. This study aims to have a better understanding of the factors that influence the decision to embark on an entrepreneurial project and to succeed in this field.
Entrepreneurial success cannot be reduced to a simple set of technical skills. Beyond skills, it is increasingly recognized that behavioural, identity and relational skills play an essential role. Entrepreneurs must be motivated, have leadership skills, and be able to effectively manage human resources, among other skills. However, the relationship between these skills and their impact on entrepreneurial motivation and success remains complex and relatively unexplored.
This study takes a comprehensive approach using various analytical methods, including Principal Component Analysis (PCA), Hierarchical Ascending Classification (HCA), and Bayesian analysis, to explore these relationships. The PCA results highlight the importance of “Know-Being” in entrepreneurial motivation. The HCA identifies five distinct profiles among potential entrepreneurs who have tested the Skills and Entrepreneurial Skills Assessment (BCAE) created by Xavier Delaunay of Pépinium . Each of these groups has specific strengths and weaknesses in terms of skills and motivation. Bayesian analysis, meanwhile, reveals complex causal relationships between the skills of “Know-Being” and “Know-How”, highlighting the impact of behavior on motivation and entrepreneurial skills.
All of these results offer valuable information for business support professionals, educators and decision-makers, highlighting the need to take into account both the behavioural and technical dimension in training and support for aspiring contractors . They also highlight the diversity of profiles among potential contractors, thus offering avenues for customizing support programs.
In short, this study contributes to our understanding of the factors underlying the motivation and development of entrepreneurial skills. It highlights the importance of cultivating both “Know-Being” and “Know-How” to promote success in entrepreneurship.
By exploring these complex relationships, we hope to provide opportunities to support next generations of contractors in their quest for success.
1. Methods and results
To better understand the relationship between “Know-Being” and “Know-How” among potential contractors, our study followed three statistical analysis: the Principal Components Analysis (PCA ), the Hierarchical Classification Analysis (HCA) and the Bayesian analysis .
As part of our study, we’ve processed a sample of 1029 potential contractors, all through the SESF questionnaire (“Know-Being” and “Know-How”) created by Xavier Delaunay from Pepinium as part of a Skills and Entrepreneurial Skills Assessment (BCAE). Excluding socio-demographic questions, this questionnaire includes 22 questions, including several questions covering different dimensions around the “Know-Being”, “Know-How” and the “motivation” of project leaders.
The questionnaire contains several sets of items covering the following different dimensions:
Motivations (8 items) |
Know-How: |
|
Business management (6 items) |
||
Know-Being: |
Sell (8 items) |
|
Self-management (6 items) |
Turnover Management (6 items) |
|
Intuition, Vision (6 items) |
Account management (7 items) |
|
Leadership (5 items) |
Managerial experience (1 item) |
|
Human resources management (7 items) |
✓ The dimensions "Know-Being" are measured on a scale of 1 = I really can not (block) -> 8 = This is my excellence, I maintain it assiduously.
The Manager experience is measured on a binary scale 1=yes / 2=no.
✓ The "Know-How" dimensions are measured on a scale of 1 = I don't know the subject -> 8 = Expert, able to advance the technique.
✓ The dimensions "motivation" are measured on a scale of 1 = I hate (or I make a real block) -> 8 = It is really a very deep desire to realize myself.
The dimensions are summarized as aggregates. For each respondent, we used here the average of the evaluations for each dimension. These summary data, for each respondent, were used in the rest of our analyses.
1.1 The first analysis we performed on this dataset is a Principal Component Analysis (PCA). PCA is a powerful statistical technique that reduces the dimensionality of data while preserving most of their variance, that is, most of the information contained in the data source. In this case, the PCA allows us in addition to expurgating the intrinsic noise from our dataset. In the social sciences’ world, data very commonly contain noise that can harm analysis.
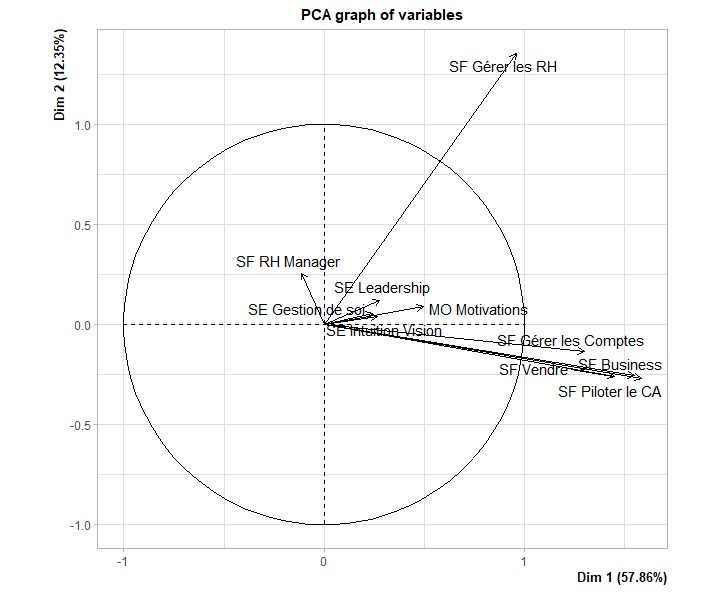
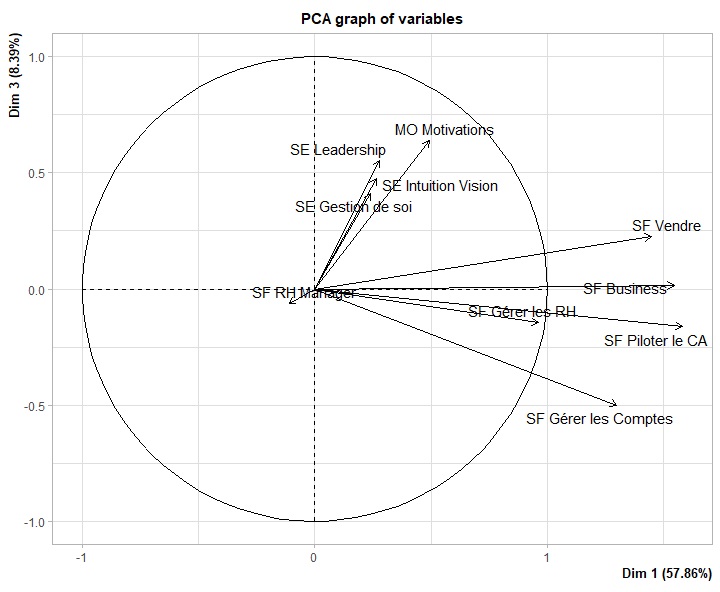
This first step clearly showed a strong multi collinearity between different items within each of the studied dimensions. The analysis also reveals a relative independence between the “Know-Being” and “Know-How” dimensions.
Thus, of the first three main components, returning 79% of the original information contained in the data, we can note the following points:
1. The different dimensions of "Know-How" are strongly correlated with each other, except "Human Resources Management", and are therefore relatively independent of the dimensions of "Know-Being".
2. "Human Resources Management" is independent of other "Know-How" skills but also of "Know-Being" skills.
3. The different dimensions of "Know-Being" are strongly correlated with each other as well as with motivation, but are independent of the dimensions of "Know-How".
1.2 The second analysis, the Hierarchical Classification Analysis (HCA), is in the partitioning register. Data partitioning is a method of the data analysis universe. It aims to divide a dataset into different groups of highly homogeneous individuals, while maximizing variance between identified groups. The individuals in each subset share common characteristics. These groups most often use a criterion of distance between individuals: similarity or dissimilarity . We have here used the Ward criterion on a squared Euclidean distance.
To achieve good partitioning, we have to:
1. Minimize INTRA-class inertia to obtain the most homogeneous groups possible;2. Maximize INTER-class inertia to obtain well differentiated subsets.
The HCA performed on the first 3 main components of the PCA at point 1.1 grouped respondents into separate groups based on their competency profiles. These results highlight five groups, each with specific profiles:
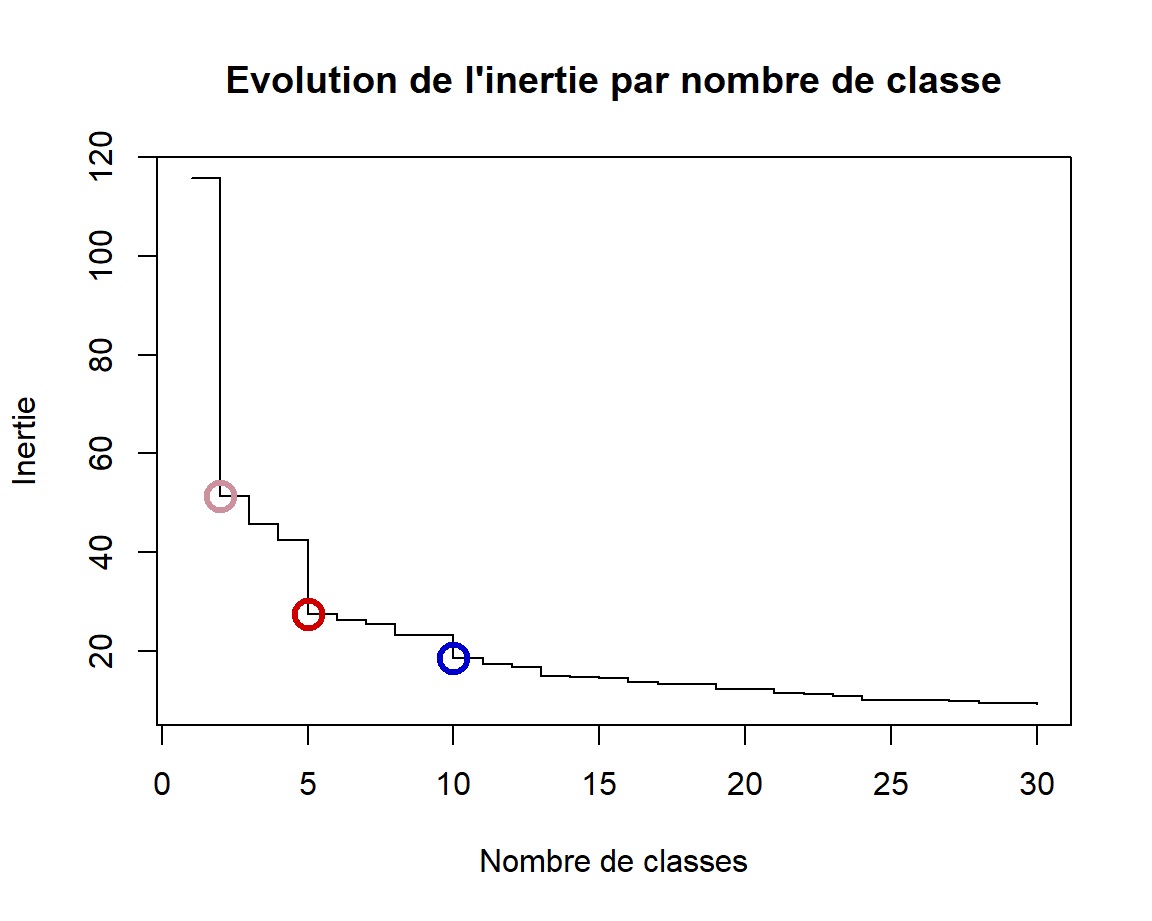
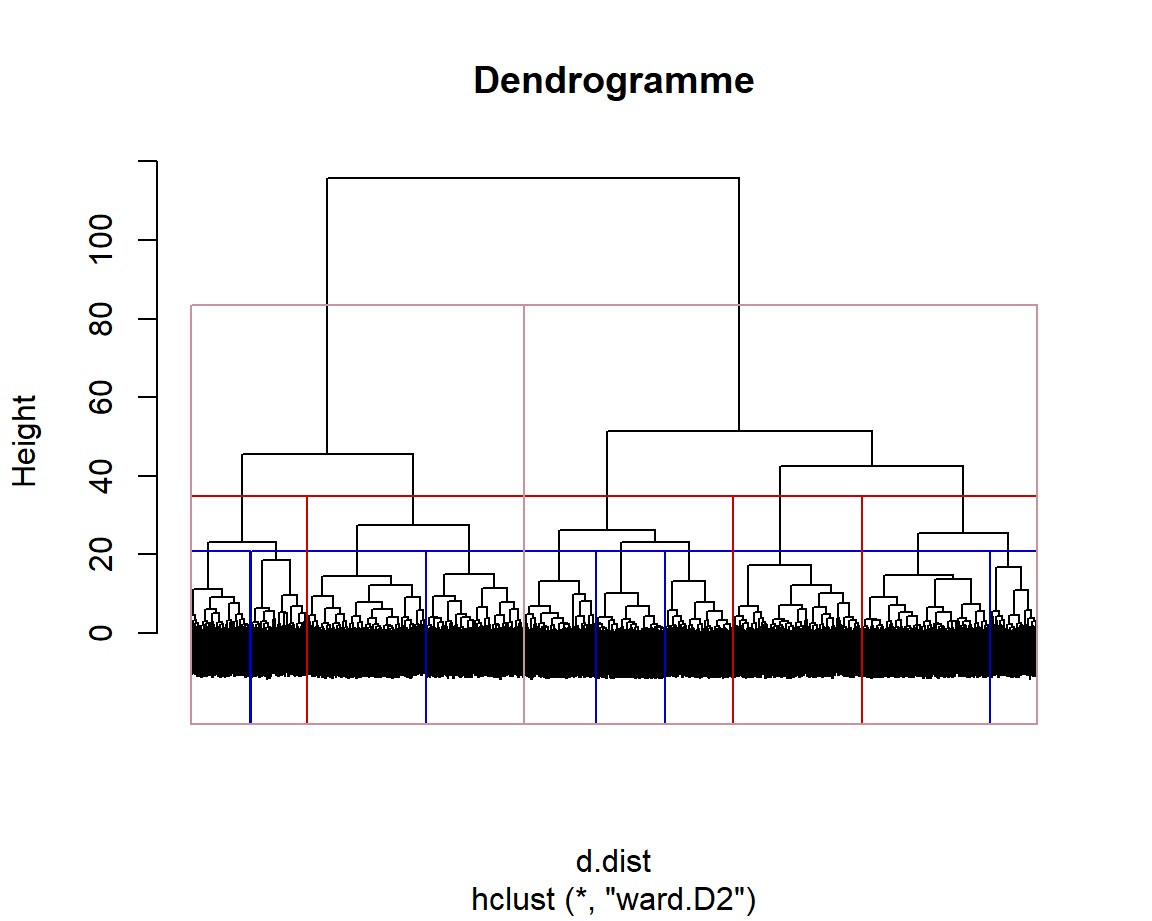
Variable | Label | Group 1 | Group 2 | Group 3 | Group 4 | Group 5 |
---|---|---|---|---|---|---|
MO | Motivations | 5.3 | 5.8 | 5.6 | 6.2 | 5.0 |
SE02 | Self-management | 6.0 | 6.2 | 6.1 | 6.5 | 5.9 |
SE03 | Intuition Vision | 6.0 | 6.2 | 6.2 | 6.6 | 5.9 |
SE04 | Leadership | 6.1 | 6.6 | 6.3 | 6.7 | 6.1 |
SF02 | Business | 2.3 | 4.2 | 3.1 | 5.9 | 1.5 |
SF03 | Sell | 3.2 | 4.9 | 4.1 | 6.4 | 2.1 |
SF04 | Turnover Management | 2.6 | 4.2 | 3.2 | 6.3 | 1.5 |
SF05 | Accounts management | 2.9 | 4.1 | 3.1 | 5.1 | 1.7 |
SF06 | Human resources management(1=yes, 2=no) | 1.6 | 1.2 | 1.0 | 1.2 | 1.4 |
SF07 | HR management | 5.4 | 5.1 | 2.6 | 5.8 | 3.0 |
except Human Resources Management (1=yes | 2=no)
In color, the items characterizing more or less significantly the group.
Red = strengths | Black = mean/median scores not significant in class | Blue = weaknesses
1. Pro-HR motivated to develop = Group 1 (20.7%): This group is mainly characterized by strong leadership, intuition/vision, human resources management ability, and motivation. However, they need to work on all other aspects of the "Know-How": Business, Drive Sales, Manage Accounts and Sell.
2. Know-how to develop = Group 2 (25.6%): The members of this group stand out by their "Know-Being", with significant motivation. However their level of "Know-How" is a bit average. Their current level leaves room for potential skills gains. Through training and/or targeted support, these respondents are serious candidates for entrepreneurship.
3. Vigilant managers = Group 3 (15.3%): This group is distinguished mainly by its lack of human resources management skills although all individuals in this group have all had experience in human resources management. The other items have no significant influence on this group. Indeed, these respondents are average or even lacking in skills on all measured dimensions. These individuals require almost complete support. They lack "Know-How" skills and their "Know-Being" is also set back from other groups.
4. Autonomous potentials = Group 4 (13.8%): Respondents in this group are distinguished by a highly developed "Know-Being" AND solid "Know-How" on all measured dimensions. They are also the most motivated of all, which suggests they are ready to embark on an entrepreneurial project.
5. Risk-motivated Know-Being without HR skills = Group 5 (24.7%): This group shares similar characteristics with Group 1, such as strong leadership, intuition/vision, and high motivation with a good ability to manage. However, they need to work on all the skills of the "Know-How", and in particular the management of human resources that should be well supported according to the entrepreneurial project of the project leader.
From this classification, we have created a predictive model allowing to reclassify in one of the five groups identified in our analysis, the future participants of the BCAE program via the SESF questionnaire. From a subset of the SESF survey data, our model affects contractors candidates in one of the previous five groups, with a correct reallocation rate of 89%. Knowing the membership of one of our 5 groups is important information and is very valuable to help actors of entrepreneurial support in their mission .
1.3 The 3rd and last statistical tool used is a Bayesian analysis.
Bayesian inference is a method of statistical analysis by which the probabilities of various hypothetical causes are computed from the observation of known events. It relies mainly on the Bayes theorem.
The Bayes theorem states conditional probabilities of several events. In its 1 763 formulation, the theorem was stated as follows:
P(A|B) =
provided that P(B) ≠ 0, and where :
- A and B are two events,
- P(A) and P(B) are the probability of both events,
- P(A | B) is the conditional probability that event A will occur since event B has occurred,
- P(B | A) is the conditional probability that event B will occur since event A has occurred.
Bayesian analysis applied to our dataset builds a causal network that provides important information about the relationships between our different dimensions.
Bayesian analysis reveals a complex causal network, highlighting a “Know-Being” impacting motivation, and indirectly the “Know-How”.
Self Management is apparently an important dimension, the source of skills to impact the other dimensions of “Know-Being”, and indirectly impact motivation and account management in the “Know-How” universe.
In the same spirit, Business Management is a fundamental skill that impacts all other skills of the “Know-How” dimension.
These relationships highlight the impact of behavior on motivation and entrepreneurial skills.
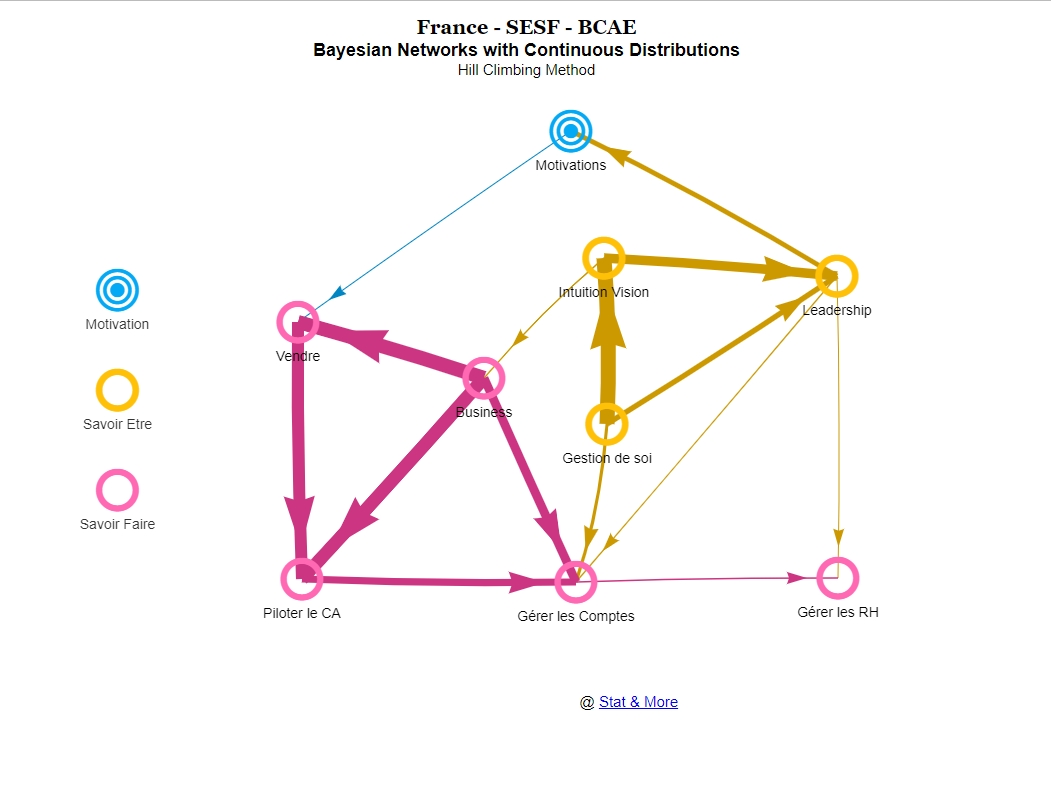
In detail:
1. The "Know-Being" items seem relatively independent of the "Know-How" items.2. "Know-Being": Self-management directly impacts intuition/vision, leadership, and in terms of "Know-How", account management.
3. "Know-How": The business dimension directly impacts the items "Sell", "Manage turnover", and the management of accounts, which in turn also impacts human resources management.
4. Motivation is impacted by leadership, and in turn, motivation impacts the "Sell" dimension.
5. The item "Leadership" also impacts human resources management.
6. The human resources dimension does not impact any other dimension. This HR dimension is impacted by account management and leadership.
The relationships between the different dimensions studied are tenuous. But when they exist, they are always oriented from “Know-Being” to “Know-How” or “Know-Being” to “motivation”, or from “motivation” to “Know-How”.
It is remarkable to note that no relationship exists from “Know-How” to “Know-Being” or “motivation”.
2. Discussion
The analysis of the results of the PCA, the HCA and the Bayesian analysis reinforces our understanding of the importance of the balance between “Know-Being” and “Know-How” among potential contractors. The Bayesian network highlights an essential “Know-Being” because it directly influences motivation, leadership, and intuition/vision, which are crucial factors for entrepreneurial success.
The link between “Know-Being” and the “business” dimension of “Know-How” underlines the importance of developing a complete set of entrepreneurial skills. The causal relationships between leadership, motivation, human resources management, and account management highlight the complexity of the factors that influence the decision to embark on an entrepreneurial project.
The results of our analysis confirm the importance of “Know-Being” and its direct impact on the motivation of individuals to undertake . This observation joins the previous work of various specialists such as Fayolle, Schmitt, Verzat, or Toutain, who highlighted the impact of so-called “soft-skills”, or “Know-Being”, on entrepreneurial efficiency. It is clear that the behavioural and identity dimension plays a crucial role in the decision to embark on an entrepreneurial project.
The groups identified by the HCA highlight the diversity of profiles among potential contractors. Group 4, balanced in terms of “Know-Being” and “Know-How”, represents an ideal model for entrepreneurship . Further analysis of this group would be beneficial to understand in detail the characteristics that distinguish it from other groups. Are their socio-demographic profile, their career path typical of this group specifically?
Bayesian analysis reveals that “Know-Being” acts as an engine. This engine directly impacts motivation, leadership, and intuition/vision through Self Management. This reinforces the need for a comprehensive (or integral) approach to supporting entrepreneurial success.
To go further in this analysis, it would be interesting to collect longitudinal data. These complementary data would allow us to follow the respondents of the SESF questionnaire over time, and to understand how all the dimensions studied impact the chances of success of the life of an entrepreneurial project. It would be particularly interesting to be able to follow in time:
1. Possible training followed by the contractor before a business creation,2. If the contractor has actually completed his business creation project, and if so:
✓ If the company created is doing well at 1, 3, 5 and 10 years,
✓ The difficulties encountered by the contractor on the dimensions measured in the SESF questionnaire in the first year of life of the company, then in the 3 and 5 years.
✓ Any training taken during the first 1, 3 and 5 years of existence of the company created.
Conclusion:
In conclusion, this summary of our analyses underlines that before “Know-How”, it is essential to “Know-Being”.
The results of this study highlight the crucial importance of “Know-Being” and its impact on the motivation and development of entrepreneurial skills among potential contractors. These analyses conducted here, the Principal Component Analysis (PCA), the Hierarchical Classification Analysis (HCA), and the Bayesian analysis, converge to reveal behavioural and identity skills essential in the decision to embark on an entrepreneurial project.
The causal network resulting from Bayesian analysis emphasizes that “Know-Being” acts as an engine, directly impacting motivation, leadership, and intuition/vision, which are key factors for entrepreneurial success. Moreover, it is clear that the “Know-How” in business management is inextricably linked to the skills of “Know-Being”. Entrepreneurial skills cannot simply be reduced to a set of technical skills, but must include behavioural and relational aspects.
The analysis of the five groups of respondents identified by the HCA reveals the diversity of profiles among potential entrepreneurs. Group 4, characterized by a balance between “Know-Being” and “Know-How”, with strong skills and strong motivation, stands out as an ideal model for entrepreneurship. A more in-depth study of this group could offer valuable information for designing more targeted coaching programs.
Ultimately, it appears from this study that to initiate an entrepreneurial project, it is essential to cultivate both “Know-Being” and “Know-How”. The development of behavioural and identity skills must be integrated into entrepreneurial support programs to promote the motivation and success of entrepreneurs. This global approach recognizes that before “Know-How”, you need “Know-Being”.
These results have significant implications for contractors, educators and decision-makers, highlighting the need to take into account both the behavioural AND technical dimension in training and support for future contractors.
Finally, the predictive model identified from the SESF questionnaire allows the actors of entrepreneurial support to operate the right levers so that each contractors positions himself in a favorable position for the creation of their company. However, neither this study nor this predictive model can presuppose the chances of success of the entrepreneur-to-be in his project, nor in the future of the company thus created.
Bibliographical References/ Webography :
Alston, C. L., Mengersen, K. L., & Pettitt, A. N. (2012). Case Studies in Bayesian Statistical Modelling and Analysis. John Wiley & Sons.
Barès, F. (2013). Dynamique entrepreneuriale Le comportement de l’entrepreneur, Alain FAYOLLE et Jean-Michel DEGEORGE, Bruxelles, De Boeck, 2012, 288 p. Revue Internationale PME, 26(1), 141. https://doi.org/10.7202/1024274ar
Bayad Mohamed, Gallais Marie, Marlin Xavier et Schmitt Christophe, « Entrepreneuriat et TPE : la problématique de l’accompagnement », https://www.cairn.info/revue-management-et-avenir-2010-10-page-116.htm
Box, G. E. P., & Tiao, G. C. (2011). Bayesian Inference in Statistical Analysis. John Wiley & Sons.
Chaffik Bakkali, Karim Messeghem, Sylvie Sammut, Les structures d’accompagnement à la création d’entreprise à l’heure de la gestion des compétences, Dans Management & Avenir 2010/9 (n° 39), pages 149 to 162, Editions Management Prospective Editions
Christel Tessier Dargent. Les entrepreneurs par nécessité : d’une dichotomie simplificatrice à un continuum complexe : définitions et typologie des entrepreneurs par nécessité : étude de la dimension effectuale des processus de création par nécessité. Gestion et management. Université Grenoble Alpes, 2015. Français. ffNNT : 2015GREAG002ff. fftel-01286640ff
Christophe Schmitt, Denis A. Grégoire. La Cognition entrepreneuriale. Enjeux et perspectives pour la recherche en entrepreneuriat. Revue de l’Entrepreneuriat, 2019, 18 (1), pp.7-22. ff10.3917/entre.181.0007ff. ffhal-02365530
Durand T. (1997), Savoir, savoir-faire et savoir être : repenser les compétences de l’entreprise, Actes de la Conférence AIMS, Montréal. Canada.
Eric Michaël Laviolette, Christophe Loue, Les compétences entrepreneuriales : définition et construction d’un référentiel – Advancia - Paris, France - 8 ième CIFEPME - Fribourg
Fayolle, A. and Gailly, B. (2009) Evaluation d’une formation en entrepreneuriat : prédispositions et impact sur l’intention d’entreprendre, Management (Paris, France : 1998), 12(3), pp. 176203.
Fayolle, Alain, Caroline Verzat. Pédagogies actives et entrepreneuriat : quelle place dans nos enseignements ? Revue de l’Entrepreneuriat 8 (2009): 1-15.
Frédérique Alexandre-Bailly. L’autonomie des individus dans l’entreprise : essai de conceptualisation et regard sur les pratiques. Gestion et management. HEC PARIS, 2001. Français. ffNNT : 2001EHEC0076ff. ffpastel-00918740ff
Gelman, A., Carlin, J. B., Stern, H. S., Dunson, D. B., Vehtari, A., & Rubin, D. B. (2013). Bayesian Data Analysis, third edition. CRC Press.
Javier Alfonso Rodriguez-Escobar, Raphaël Lissillour et Marie-José Scotto, « Formations à l’entrepreneuriat : les connaissances aptes à développer l’intention d’entreprendre », Formation emploi [En ligne], 160 | Octobre-Décembre, mis en ligne le 05 janvier 2024, consulté le 11 janvier 2024.
Julien de Miribel, Xavier Sido. Définir et reconnaître les compétences entrepreneuriales ? [Rapport de recherche] Rectorat de l’Académie de Lille, IDEE. 2019. ffhal-03661504ff
Khairi Mohamed Omar, Measuring the Entrepreneurship Characteristics and Its Impact on Entrepreneurial Intentions, Open Journal of Business and Management > Vol.9 No.2, March 2021
Laviolette EM., Loue C., « Les compétences entrepreneuriales : définition et construction d’un référentiel», 2006
Lebart, L., Morineau, A., & Piron, M. (2000). Statistique exploratoire multidimensionnelle.
Le Pontois Sandrine, « L’impact de l’éducation en entrepreneuriat au prisme de son évaluation : pour une approche multidimensionnelle de l’efficacité de l’éducation en entrepreneuriat », 2020, https://theses.hal.science/tel-03275765
Maindonald, J. (2011). Exploratory Multivariate Analysis by Example Using R by François Husson, Sébastien Lê, Jérôme Pagès. International Statistical Review, 79(3), 498. https://doi.org/10.1111/j.1751-5823.2011.00159_19.x
Marin, J., & Robert, C. (2013). Bayesian Essentials with R. Springer.
Mégret, J.-M. (2022) Eduquer à l’esprit d’entreprendre: entre enjeux éducatifs et politique éducative, regard sur la recherche, Projectics, 32(2), pp. 3758.
Naffakhi, H., Boughattas-Zrig, Y., & Schmitt, C. (2008). La création de connaissances au niveau des équipes entrepreneuriale. Revue de l’Entrepreneuriat, 7(1), 23.
Qiong Gui*, Wenqing Chen, Xuming Zhang, Impact of Entrepreneurial Self-Construction on Entrepreneurial Intention: Moderating Role of Institutions, Journal of Human Resource and Sustainability Studies > Vol.9 No.2, June 2021
Schmitt Christophe, Rosker Eduardo, « Quand savoir entreprendre, c’est savoir concevoir », La Revue des Sciences de Gestion, 2015/3-4 (N° 273 - 274), p. 71-82. DOI : 10.3917/rsg.273.0071. URL : https://www.cairn.info/revue-des-sciences-de-gestion-2015-3-page-71.htm
Wang, Y. (2010). L’évolution de l’intention et le développement de l’esprit d’entreprendre des élèves ingénieurs d’une école française : une étude longitudinale. https://www.theses.fr/2010ECLI0011.pdf
Wang Yifan, «L’évolution de l’intention et le développement de l’esprit d’entreprendre des élèves ingénieurs d’une école française : une étude longitudinale», 2010, https://www.theses.fr/2010ECLI0011.pdf.
Wang Yifan, Bigand Michel et Frugier Dominique, « Comment transformer les futurs ingénieurs en entrepreneurs ? », dans Entreprendre & Innover, 11-12, no 3, 1 janvier 2011, p.65, https://doi.org/10.3917/entin.011.0065.
Yvon Pesqueux. De l’entrepreneur et de l’entrepreneuriat. Doctorat. France. 2024. ffhalshs-02915781v4ff
Zaki, M. J., Meira, W., Jr, & Meira, W. (2014). Data Mining and Analysis: Fundamental Concepts and Algorithms. Cambridge University Press.